Editor’s note: Supply Chain Management Review is launching a series of new online features in 2024, including The Academy. Appearing online at scmr.com on the third Wednesday of each month, the Academy will look at education within the supply chain. If you are interested in future topics, you can see a full list of upcoming topics on our Editorial Calendar.
In supply chain higher education, students are learning to use Generative Artificial Intelligence (GenAI) like ChatGPT. As they gain more experience with the technology, habits, expectations, and GenAI best practices emerge. Those norms will follow our graduates into the workplace as supply chain organizations hire them. Executives should know how GenAI is being used in supply chain higher education to better understand what capabilities new graduates may bring to the table.
By making artificial intelligence more accessible to non-data scientists, GenAI has the potential to enhance productivity, alter processes, and enhance relationships between supply chain partners. Disruptive technologies emerge consistently, but not since the internet has there been one that so drastically impacts teaching and learning. The GenAI best practices in supply chain education are emerging. To translate them into practical guidance to realize the potential, industry and academia must form partnerships to study the technology in the field.
GenAI in industry
According to a recent Gartner poll, nearly half of the surveyed companies plan to invest in GenAI soon. Considering that Microsoft now offers Copilot as part of its enterprise suite of products, the pace of GenAI adoption in supply chain management will likely accelerate. As with any novel technology, the key question of “how will it create supply chain value?” remains to be answered. Supply chain managers see new technologies emerge regularly, promising results from disruption to revolution. Often, the hyperbole fades as such technologies fail to deliver lofty expectations, leading to hesitation or skepticism.
Supply chain organizations are already embracing GenAI.
GenAI has a similar perception, which, to be frank, is warranted since even cutting-edge models like OpenAI’s GPT-4 suffer from hallucinations (note: GPT-4 has one of the lowest hallucination rates as of this writing, according to the Hughes Hallucination Evaluation Model (HHEM) leaderboard). These risks are areas of ongoing research, and future breakthroughs may reduce occurrence rates. However, whether these hallucinations can be eliminated is an open question since what makes LLMs hallucinate is also what makes them generate crafty responses.
GenAI in supply chain academia
The hesitation around GenAI in higher education stems partly from concerns about academic misconduct. A common policy is to ban the technology outright from the classroom. AI detectors may be used to judge whether a student’s work is original or AI-generated, but such tools are unreliable. For this reason, OpenAI discontinued its own AI detector, and some detection companies like Originality.ai even recommend against using them in academic misconduct investigations. Studies have also shown that detectors are biased against non-native English writers. Given the rise of tools that enable AI-generated content to be rewritten in a more “human” tone that passes AI detection, it is apparent that prohibiting GenAI in the classroom leads to an arms race that fosters mistrust between students and professors. This environment also creates a scenario where students cannot experiment with AI and, therefore, cannot learn when and when not to trust it. Simply put, those students are entering the workforce at a disadvantage if they don’t understand what AI can and cannot do.
On the other hand, an open culture promoting transparent and responsible use of GenAI will help students learn about the technology’s benefits and limitations. I encourage my students to use GenAI on their assignments and share feedback about their use. In fact, I even require them to state whether they’ve used it and to provide a link to the GenAI conversation thread that documents their interactions.
Requiring this transparency about GenAI use serves two purposes. First, it brings usage into the light, making misconduct less likely since students know I’m going to read their threads. Second, accessing their threads allows me to give feedback to improve their prompt engineering skills. In class, I’ve found that the prompt is usually the culprit when the GenAI produces a poor response. This leads to an important teaching moment – GenAI is pretty good at guessing your intent, but you must help it help you. That means finding the right terminology or combinations of words that enable GenAI to generate relevant responses that match your prompt.
Responsible use also requires learning about the risks of GenAI and why they occur – hallucinations and plagiarism can be traced back to how GenAI remixes the patterns found in training data (this is an overly simplified explanation, of course). AI hallucinations are similar to humans seeing objects in the clouds. We see patterns in clouds that remind us of images we’ve seen in the past, but of course, they’re not actually there. GenAI exhibits the same phenomenon by trying to reproduce patterns found in its training data based on what it “sees” in the prompt. This discussion helps students understand that their prompts may lead to a greater hallucination risk when not carefully crafted.
With these policies and ongoing dialogue undergirded by transparency and responsibility, I aim to foster openness around AI. Such norms allow students to ask questions and experiment with different applications of AI freely. With any luck, these norms will follow my students into the workplace so that they can innovate with GenAI to create supply chain value. Doing so will require learning new AI skills.
Introducing Supply Chain Brutus
GenAI allows for innovation in supply chain education that leads to those new skills. Using the new GPTs feature from OpenAI, I have created an AI-based tutor called Supply Chain Brutus (note: you’ll need a ChatGPT Plus subscription to access it). GPTs are customized versions of ChatGPT for specific use cases. Users instruct GPTs in natural language prompts and can fine-tune their GPT by uploading additional files.
(Figure 1 - Supply Chain Brutus. Image created with the assistance of DALL E-3, OpenAI (2023)).
Supply Chain Brutus is a GPT fine-tuned on the content of my supply chain analytics course. Fine-tuning means refining the base GenAI model with specific knowledge to improve its performance in a particular domain. I have uploaded a copy of my working textbook and course notes to expand its knowledge base with my curriculum. Having instructed it how to behave using plainly written instructions (e.g., “Do this, don’t do that”), Supply Chain Brutus acts like an upbeat tutor to help students learn the fundamentals of analytics. This resource also shows students how they can tailor GenAI for specific use cases. Recently, one of my students showed me their GPT where the user describes a product being imported, and the GPT returns a list of codes in the Harmonized Tariff Schedule (HTS) that most closely match the product description.
A call to action for industry and academia
When I meet with industry executives to learn about supply chain analytics in practice, I often hear about a communications problem – the experts with the skills to produce rigorous analytics models may not have the first-hand expertise with the supply chain processes to be analyzed and that managers who do have that first-hand expertise, don’t have deep operations research knowledge. The result can be two groups of experts struggling to understand each other. GenAI can help bridge this communications gap to improve the managerial insights gained from analytics.
How GenAI will bridge that knowledge gap remains to be seen, but industry-academia partnerships can jumpstart the process. By testing and studying GenAI applications in the classroom, the best cases for creating true supply chain value will emerge.
This article was written without the assistance of generative AI.
About the author
Vince Castillo, Ph.D., M.B.A. is an assistant professor of logistics at the Fisher College of Business at The Ohio State University. His research interests include last mile logistics, supply chain sustainability, simulation modeling, and artificial intelligence. Professor Castillo holds a PhD in Supply Chain Management from the University of Tennessee (2018), an MBA in Supply Chain Management and Finance (2014, University of San Diego), and a BS in Civil Engineering (2006, Colorado School of Mines). Dr. Castillo is also a GWOT veteran, having served as an Infantry Officer in the Army National Guard from 2006-2014.
SC
MR


Latest Supply Chain News
- Open eBL: Transforming the electronic bill of lading with open-source innovation and industry collaboration
- GenAI’s role in advancing supply chain planning
- August retail sales are up, notes Commerce and NRF
- Will the new president of Mexico help or hinder manufacturing?
- A scheduled workforce is a stable workforce
- More News
Latest Podcast
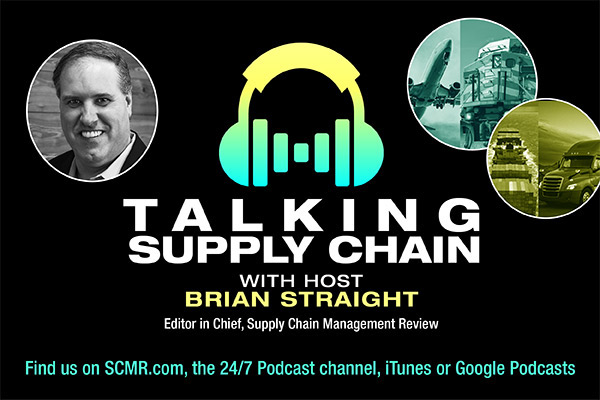
Explore
Education News
- 2024 Robotics Application Conference announces session, speaker lineup
- NextGen Supply Chain Conference announces Solution Provider Award winners
- Join the Real Housewives of Supply Chain, change leaders in exclusive sessions at NextGen Supply Chain Conference
- The benefits and challenges of RPA in logistics
- Learning from the past to invent the future of last-mile logistics
- Squint named NextGen Supply Chain Conference Start-Up Award winner
- More Education
Latest Education Resources
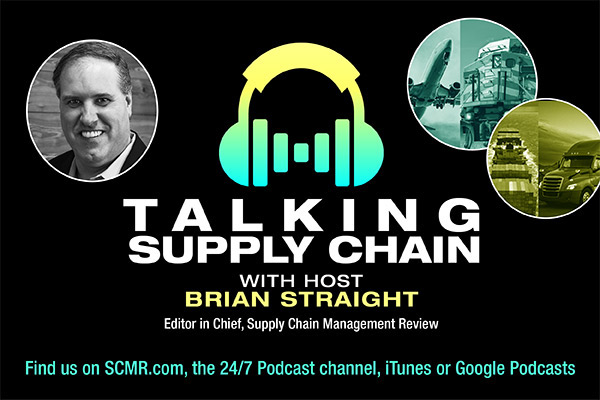
Subscribe

Supply Chain Management Review delivers the best industry content.
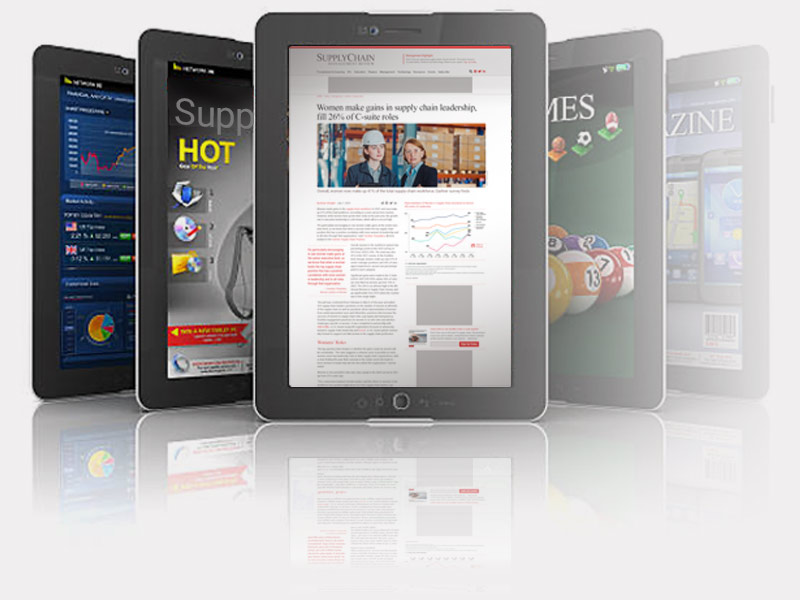
Editors’ Picks



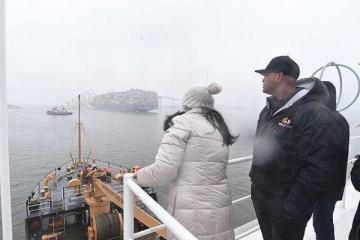